ICAMS Fire Workshop III
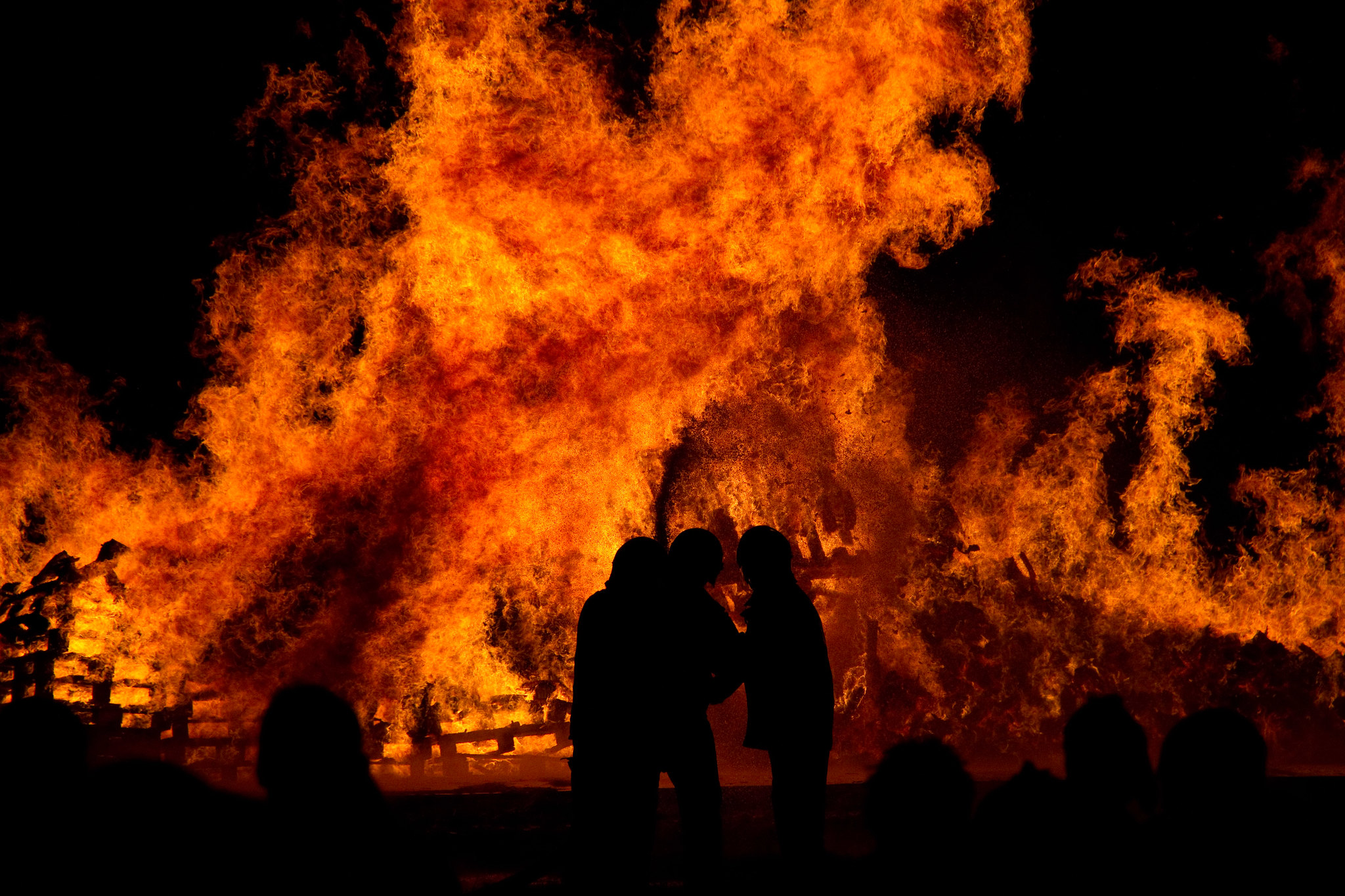
Fire. Image by Tiago Cabral, Flickr.
The third ICAMS Wildland Fire Workshop
The third ICAMS Wildfire Workshop took place on October 12th in full virtual format over zoom.
The Interagency Council for Advancing Meteorological Services (ICAMS) is the principal means within the Executive Branch to coordinate priorities across the diverse agencies that make up the Federal meteorological services enterprise.
Wildland fire science is a multifaceted discipline. As such, this workshop was focused on research and applications of Subseasonal to Seasonal (S2S) (2 weeks to 2 years) forecasting for wildland fires. The workshop agenda included invited talks, discussing the current state of S2S predictive services, applications, and research that are applicable to wildland fire prediction, as well as more focused discussions during two breakout sessions.
Scott Weaver, director of the Interagency Meteorological Coordination Office (IMCO, the administrative headquarters of ICAMS providing logistical and other support), set the stage for the workshop by describing the origin of ICAMS as fulfilling the mandate of the 2017 Weather Research and Forecasting Innovation Act to improve coordination of relevant weather research and forecast innovation activities across the Federal Government. Dr. Weaver closed his talk by describing S2S diagnostics and prediction factors that relate to ENSO, the MJO, and the Drought Monitoring.
Rich Naden (National Park Service/Predictive Services) presented “Longer-term forecast products—what, how, and why predictive services use information for various purposes associated with fire management.” He noted that the capability to use S2S forecasts is not built into fire prediction and is a primary limitation or obstacle to using subseasonal outlooks.
Ben Kirtman (University of Miami-Rosenstiel School) reported on “North American Multi-Model Ensemble (NMME) and Subseasonal Experiment (SubX) Projects: Informing Fire Weather Forecasts.” His presentation provided overview and current status of the SubX and the NMME projects. SubX and NMME are multi-model seasonal forecasting systems producing well-calibrated, robust estimates of future weather and they differ in the type of models used and the frequency in which they are issued. Dr. Kirtman also discussed an attempt to examine the Hot-Dry-Windy Index (HDW) in SubX and NMME that include focus on large-scale climate drivers of hyper-hyper local variability. He concluded that the HDW index may need some modifications for S2S time scales.
Robert Field (NASA Goddard Institute for Space Studies) presented “Wildland fire prediction at S2S scales”. Dr. Field opened his presentation with a review of fire fundamentals and what works in identifying fire danger. He reviewed a case study for Fire danger forecast evaluation for Alaska in 2022, which ranked #4 in the burned area since 2002. This evolution of fire activities was evaluated in terms of a Build Up Index (BUI), a numerical rating of the total fuel available for combustion. Furthermore, Dr. Field noted that a multi-model fire danger hindcast archive would be helpful for 1) comparing forecast skill across weather models, fire danger rating systems, spatial scales and temporal scales using common skill metrics; 2) building more comprehensive fire occurrence prediction models that include ignition; 3) predicting smoke emissions in chemical weather forecasts; 4) developing simplified inputs for ML approaches; and 5) using land surface models with surface data assimilation (e.g., soil moisture overwintering, dynamic fuel loads, curing) to predict flammability.
Elizabeth A. Barnes (Colorado State University) presented “Explainable AI (XAI) for State-Dependent Subseasonal-to-Seasonal Prediction (of Wildfires).” Dr. Barnes opened her talk with a statement that we cannot expect to make perfect predictions all the time and “beyond the weather timescale we must look for specific states of the earth system, i.e., opportunities, that lead to enhanced predictable behavior”. XAI can be helpful for identifying climate patterns that lead to accurate temperature predictions on seasonal timescales. For example, exploring how tropical information can lend predictability to midlatitude circulation on S2S timescales. Furthermore, ML can be applied to improve understanding of S2S predictability & prediction, including deep learning weather prediction models, improved model parameterizations, post-processing of multi-model ensembles, downscaling for regional impacts, predicting the Errors of Forecast Systems, and statistical model predictions.
L. Ruby Leung (Pacific Northwest National Laboratory) presented “Wildfire Prediction: Insights from Machine Learning Models and Observations.” Dr. Leung opened with these key messages that machine learning (ML) models and explainable AI are useful tools for understanding the drivers of wildfires and diagnosing biases in process-based fire models. She also noted that large-scale meteorological patterns, in addition to local meteorology, are important predictors of wildfires at monthly, seasonal, and interannual timescales. Dr. Leung described ML models that include large-scale meteorological patterns as predictors and explained that these ML models can capture spatial and temporal variability of wildfires. In using ML models to understand model biases in Fire modeling intercomparisons (FireMIP), the Data-driven ML model is more skillful in simulating fire emissions. FireMIP models generally display larger sensitivity to meteorology than observed or the ML model.
Invited talks were followed by two breakout sessions that focused on 1) improving the science for wildland fire prediction at S2S scales, and 2) Post processing, verification, and evaluation.
Recorded presentations, slides, and workshop summary will be soon posted online at ICAMS Wildfire Workshop (icams-portal.gov).
References
- E3SM Newsletter posts:
This article is a part of the E3SM “Floating Points” Newsletter, to read the full Newsletter check: