Coupling a Stochastic Convective Parameterization with the Zhang–McFarlane Scheme Copy
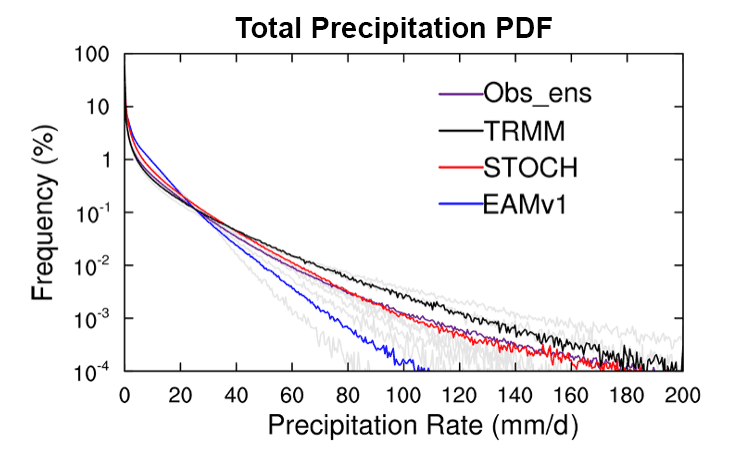
Figure 1. Frequency distributions of total precipitation intensity over the tropics (20° S–20° N). Gray lines: observation ensembles, with their mean represented by the purple line. Black TRMM line: the Tropical Rainfall Measuring Mission 3B42 version 7 data. Blue EAMv1 line: the standard EAMv1 simulation with the default deterministic Zhane-McFarlane (ZM) deep convection scheme for simulating the current climate. Red STOCH line: same simulation as EAMv1 but with the stochastic deep convection scheme coupled with the deterministic ZM scheme. The red coupled convection scheme (STOCH) matches the observation ensembles much more closely than EAMv1 with only the ZM scheme.
The “too much light rain and too little heavy rain” problem is alleviated with the use of a stochastic deep convection scheme.
The Science
Precipitation plays a vital role in the Earth’s climate: the latent heat released during precipitation formation is a major energy source that drives the atmospheric circulation, and precipitation is an important part of the Earth’s hydrological cycle. The accurate simulation of precipitation in global climate models (GCMs) is of great scientific and societal interest. However, GCMs used for current climate simulation and future projections suffer from many biases in the global distribution, frequency, and intensity of simulated precipitation, which have negatively impacted model fidelity. In this study, scientists show that GCM precipitation simulations can be significantly improved by incorporating a stochastic convective parameterization. More specifically, a stochastic deep convection scheme is coupled with the Zhang-McFarlane (ZM) deterministic deep convection scheme within DOE’s E3SM Atmosphere Mode (EAM) version 1 (v1) as shown in Figure 1.
The Impact
This study provides a better representation of convection which in turn improves the modeling of precipitation – its global and regional distribution, intensity and frequency. More accurately simulating precipitation is critical for providing realistic climate change projections for decision making and impacts applications.
Summary
By implementing a stochastic deep-convection scheme into the Zhang-McFarlane (ZM) deterministic deep-convection scheme, scientists improved the representation of convection in EAMv1. The well-known problem of “too much light rain and too little heavy rain” was alleviated, especially over the tropics. The mean precipitation amount distribution improved with more precipitation contribution from more intense precipitation events. The synoptic and intraseasonal variabilities of precipitation were enhanced and were closer to observations.
Funding
- The U.S. Department of Energy Office of Science, Biological and Environmental Research Program supported portions of this research as part of the Regional and Global Model Analysis (RGMA), Atmospheric System Research (ASR), and Earth System Model Development (ESMD) program areas.
- This research used resources of the National Energy Research Scientific Computing Center (NERSC).
Contact
- Guang Zhang, Scripps Institution of Oceanography