Significance of Improved Initialization in Climate Models for Subseasonal-to-Seasonal Precipitation Prediction
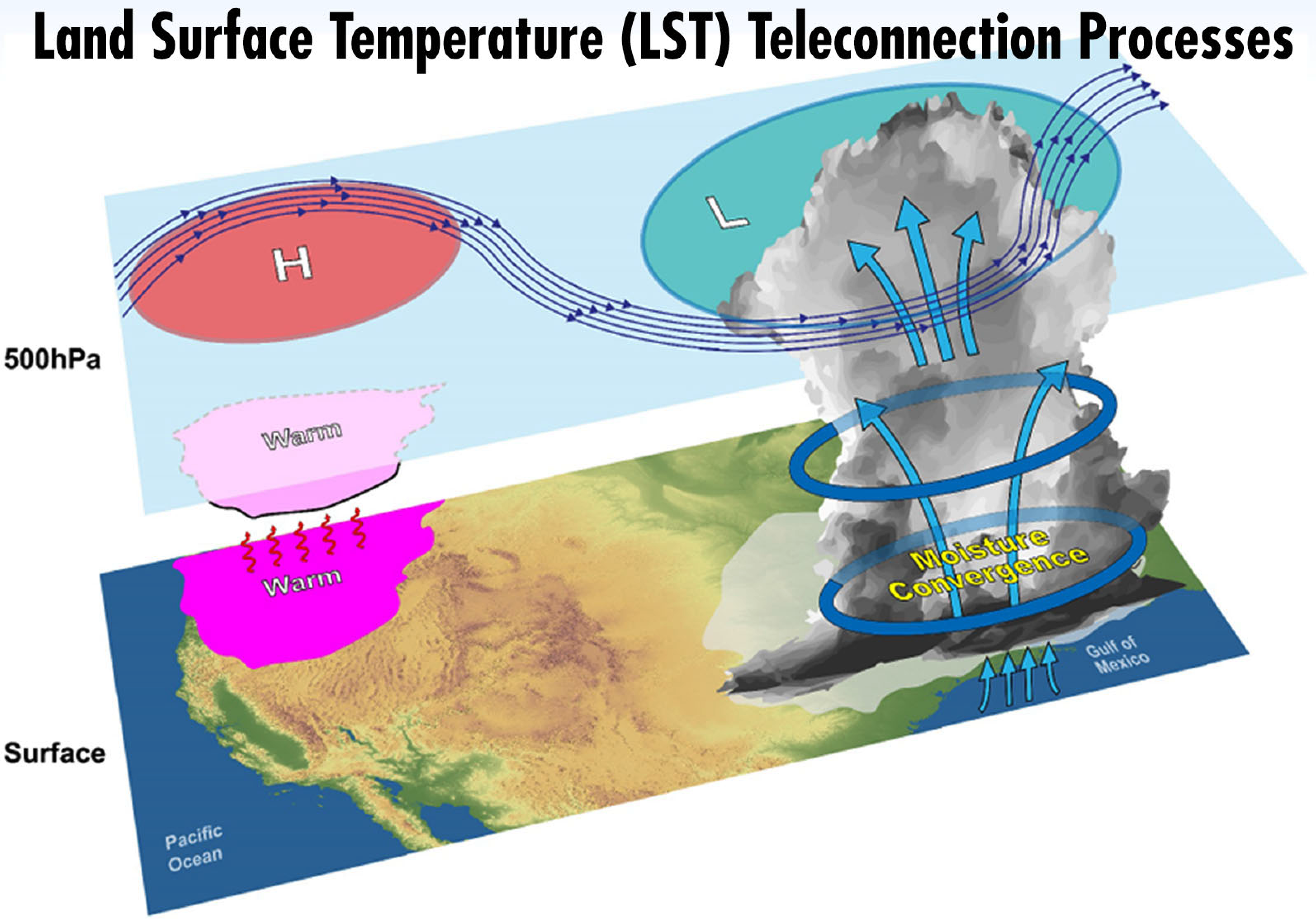
Teleconnection between spring land surface temperature and summer downstream precipitation (Figure from https://ls4p.geog.ucla.edu/)
The study finds that a data assimilation technique can create more realistic initial conditions, which are important to improve subseasonal-to-seasonal (S2S) precipitation predictions in climate models.
The Science
Reliable subseasonal-to-seasonal (S2S) precipitation prediction is highly desired due to the great socioeconomical implications, yet it remains one of the most challenging topics in the weather/climate prediction research area. As part of the Impact of Initialized Land Temperature and Snowpack on Sub-seasonal to Seasonal Prediction (LS4P) project of the Global Energy and Water Exchanges (GEWEX) program, twenty-one climate models follow the LS4P protocol to quantify the impact of the Tibetan Plateau (TP) land surface temperature/subsurface temperature (LST/SUBT) springtime anomalies on the global summertime precipitation (Xue et al. 2022).
Scientists at Lawrence Livermore National Laboratory within the Atmospheric, Earth, and Energy Division, along with collaborators from scientists from Pacific Northwest National Laboratory, University of California Los Angeles, and Tsinghua University, examined the impact of initial conditions on subseasonal-to-seasonal (S2S) precipitation prediction in two climate models, E3SMv1 and Community Integrated Earth System Model (CIESM) (Lin et al. 2020). The selection of the two models is based on their respective warm (CIESM) and cold (E3SMv1) biases in surface air temperature compared to the observation over the TP, which represent two groups of LS4P climate models that share similar biases.
The focus of this study was to reveal the importance of the nudging approach to generating more realistic initial conditions for S2S precipitation predictions.
The Impact
This study highlights the important role that initial conditions play in the S2S prediction and suggests that a data assimilation technique, “nudging”, should be adopted to initialize climate models to improve their S2S prediction.
Summary
The nudging method (Fig. 1) is a data assimilation method that uses an additional term in the model equations to drive the model towards a reference state, which can be observed data, reanalysis, or a higher resolution model result. In this research, only the horizontal winds at all vertical levels were nudged, which helps produce a more realistic initial condition of large-scale wave pattern (Fig. 2) and also generates more consistent clouds and aerosol properties. The nudging is conducted at every model timestep towards the European Center for Medium-range Weather Forecasting Interim (ERAI) reanalysis.
Figure 2. Non-zonal geopotential height (m) at 200 hPa from (a) ERAI reanalysis, (b) CIESM with nudging, (c) CIESM without nudging, (d) E3SMv1 with nudging, and (e) E3SMv1 without nudging on April 30th, 2003. The images show more realistic wave patterns after applying nudging approach, critical for S2S prediction.
Simulations with nudged initial conditions can better capture the impact of the springtime land temperature anomaly over the Tibetan Plateau on the summertime precipitation predictions, as observation showed (Fig. 3). Further analyses show that the enhanced S2S prediction skill is largely attributable to the substantially improved initialization of the Tibetan Plateau-Rocky Mountain Circumglobal (TRC) wave train pattern in the atmosphere.
Figure 3. (a )TP 2-m air temperature anomaly (K) from 1st–15th May 2003 observation, 15-day averaged 2-m air temperature responses in E3SM and CIESM simulations with nudging ICs (i.e., EXP2-NudgIC minus EXP1-NudgIC) and E3SM and CIESM simulations without nudging ICs (i.e., EXP2 minus EXP1) simulations. (b), (c) June precipitation response (mm/day) over eight hot spots. SYRB: South of Yangtze River Basin (112–121° E; 24–30° N); NEast Asia: North of East Asia (120–135° E; 40–50° N); E Africa: East Africa (27–37° E; 3°S–8° N); Sahel: Sahel (12° W–13° E; 10.5–16° N); SGP: Southern Great Plains (105–90° W; 30–40° N); Central America: Central America (110–87° W; 13–29.5° N); N South America: Northern South America (80–51° W; 4–12.5° N); and NW_US: Northwest United States (124–105° W; 45–55° N). The definitions of hotspots are referred from Xue et al. (2022).
Publication
- Qin, Yi, Qi Tang, Yongkang Xue, Ye Liu, and Yanluan Lin. 2024. “Improved Subseasonal-To-Seasonal Precipitation Prediction Of Climate Models With Nudging Approach For Better Initialization Of Tibetan Plateau-Rocky Mountain Circumglobal Wave Train And Land Surface Conditions”. Climate Dynamics. Springer Science and Business Media LLC. doi:10.1007/s00382-023-07082-1.
Funding
- Qi Tang’s work was supported by the Earth System Model Development program area of the Department of Energy, Office of Science, Biological and Environmental Research program and the Lawrence Livermore National Laboratory (LLNL) LDRD project 22-ERD-008, “Multiscale Wildfire Simulation Framework and Remote Sensing”.
Contact
- Qi Tang, Lawrence Livermore National Laboratory
This article is a part of the E3SM “Floating Points” Newsletter, to read the full Newsletter check: